The New Age of Venture Capital
Last month, a host of tech experts, entrepreneurs, and investors all came together in London for our inaugural Pulse AGM.
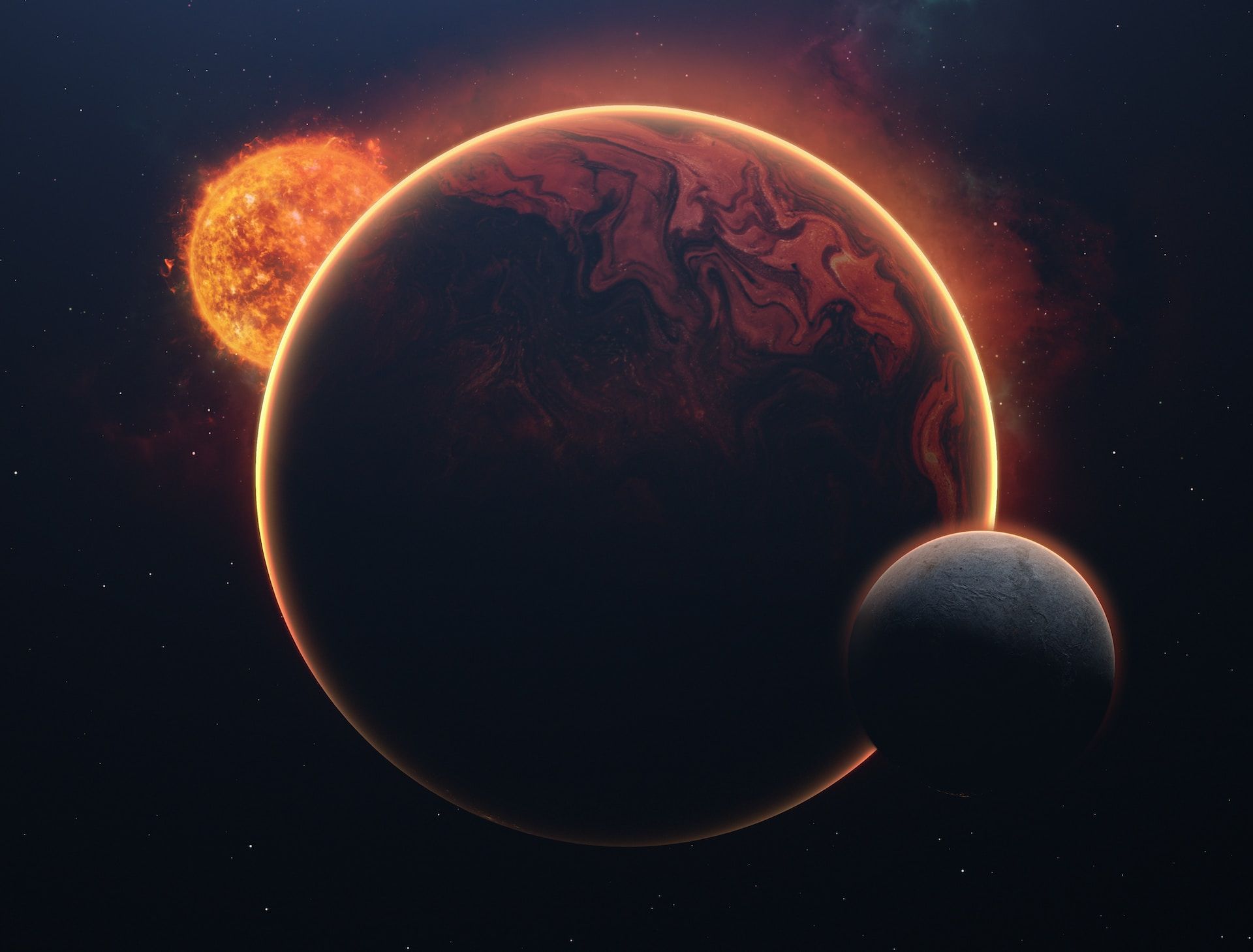
Last month, a host of tech experts, entrepreneurs, and investors all came together in London for our inaugural Pulse AGM, where our panellists discussed the ever-changing world of capital and finance, disrupting the future of work, the role of machine learning (hereafter ML) in VC, crypto in gaming, and more.
And in order to understand these technologies and our role in that vision, it is important for us to tell you about ML, and how it is shaping the future of ethical investing here at Moonfire and beyond. ML is just the beginning, and we at Moonfire will keep bringing you up-to-date content from the world of future technologies.
This is why we have officially launched our new Pulse media centre, where you can find even more great content, and sign up for further emails, including another write-up from our AGM.
We look forward to building an innovation-first future together.

Why the future of ethical investing lies in machines
For us here at Moonfire, it has always been an immense privilege to share insights with some of the most inventive entrepreneurs and tech visionaries working to transform the organisations of tomorrow.
And, following last month’s (May’s) successful AGM – known as Moonfire Pulse – where experts, investors, and founders from across the tech space gathered to exchange ideas, we are inspired anew.
Some of the key insights from Pulse will follow below, highlighting transformations in digital health and in the future of work, fintech, and gaming.
Alongside many of our partners, we are endeavouring to build the tools, environments and resources necessary to challenge the status quo. Through these efforts, we hope to push boundaries, solve problems, and achieve growth.
Our partners' experiences and learnings inform us, and, as such, help to articulate Moonfire’s own approach as a next-generation VC fund – whose passion will forever be in the creation of something greater than the sum of its parts.
To this end, we hope to add some further understanding of AI and data-driven processes here – speaking to both the value we believe our approach brings to founders as well as to the European venture space more broadly.
We’ll be writing a lot more on this topic in the coming weeks and months. In the meantime, we hope to share some understanding behind our approach.
Building operations from the ground up – Moonfire’s data-driven approach
The use of advanced data science and ML to enhance the VC process is a well-known and increasingly trodden path.
Advancements in AI, especially in the field of NLP (Natural Language Processing), where computers learn to analyse human sentiment and intention in blocks of text, have led to the development of tools capable of transcending traditional business intelligence.
These language models, when fine-tuned by humans, can greatly assist and expedite reasoning, strategic thinking and the illumination of hidden market signals.
ML is currently used in around 5% of VC firms to identify rewarding opportunities with unrivalled efficiency, with that number expected to soar over the next three years. AI can be invaluable in leveraging vast data sets, and in eliminating low or no-return startups.
Yet, typically, even in the most vociferous data-driven VC firms, this process often amounts to little more than a like-for-like investment opportunity comparison, using a single statistical model barnacled on to a still-traditional decision-making pipeline.
Moonfire’s approach lies in the development and use of advanced ML - with an emphasis on the continual data-informed honing and calibration of our models - throughout the entire venture tech ecosystem.
In short, we’re building our operations from the ground up, using technology to craft better processes that, when integrated with human insight, enable human investors to make much better decisions.
Why use ML in VC?

“We see ourselves as a tech company whose business is venture – rather than a venture firm that dabbles with tech.”
— Mattias Ljungman, founder of Moonfire, speaking at Pulse
We believe that adaptive automation will become the cornerstone of ethical investment. It reduces bias in human judgements, false assumptions and pack mentality, while shrinking information asymmetry.
Furthermore, it serves to reduce individual investor hubris, and through the acceptance of scientific principles over human cognitive bias, helps skew towards the humble.
So, rather than diminishing human relationships, ML actually elevates them by enhancing how VCs and founders can work together.
There are several ways that introducing AI and iterative algorithms into deal flow can amplify diversity and foster better relationships.
- By utilising an array of non-traditional data sets, a series of high-contextual algorithms can quickly –and with ever-increasing levels of nuance – identify promising startups that otherwise could become lost in the crowd. This process has already manifested in greater geographical and emerging market diversity, especially across a region as varied as Europe, introducing VCs to entrepreneurs they may otherwise have dismissed.
- Secondly, by factoring in and optimising for diversity within these smart tools, further guardrails can be put in place to counter the unconscious biases of VCs. Our aim has always been to make venture capital available to all the right founders – not just those who follow the traditional systems of the past. And, in doing so, we also make the process far more efficient.
- Thirdly – and to that end, one of our more bespoke approaches is to use ML to foster better alignment of VCs and founders. This innovation seeks to build better partnerships through more synchronised visions and alliances. Conversely, by avoiding pairings of misaligned partners and using software to correlate for fund values and culture, we can save both founders and VCs huge amounts of time.
So, by shifting the VC process from a mostly manual “gut-driven” approach to one incorporating science and ML, we hope to build a financial institution that will outlive our founders, their personal networks, and any software we create. And is one that keeps on improving into the future.
A ML stack for VC: (How does our approach work?)
As we have explained, it is the depth to which we use software, data and ML across all areas of our ecosystem – informing every level of the stack, and with many of the models hierarchical and feeding into each other – that brings us our distinctiveness.
Sourcing – In sourcing, our process begins by analysing the public presentation of companies or founders, using advanced natural language processing.
We analyse text across social media; product launch websites; high-quality incubators and accelerators with a strong presence in Europe – and also domain-specific indexes and aggregators too.
Some of these give us amazing APIs and data feeds, but, for others, we have to build the infrastructure ourselves to garner these inputs in a reasonable manner that’s not disruptive to the application.
Then we have to store this data, index it, aggregate it, do analysis on it, and screen it – based on sector, stage to scale, for example.
And then we use cutting-edge natural language processing to evaluate the company with respect to our natural language articulated investment thesis. Which is, I think, the most interesting, technical bit of ML that we do.
Founder Evaluation – This initial analysis scores founders and startups on a range of different attributes and dimensions, before combining these signals to calculate an aggregate score.
The combination can be a learned or a heuristic algorithm, but it’s the learning part of this process when evaluated against Moonfire’s own continually-evolving investment thesis, that really helps us decide if a founder is a good fit for us.
This data analysis also shows where certain skills combine together to be significant indicators of strength.
Moonfire currently scores for up to 50 different signals during its thematic evaluations, screening for metrics that are strongly correlated with performance, such as experience, and then metrics such as DPI, or probability and magnitude of exit.
We try to come up with as many signals as possible; as long as we can do some statistics to indicate that they add some correlative value. And then we concatenate them all together into a vector, to be then fed into a ML model which tries to learn relationships between them.
Alignment with Moonfire – Other signals in the mix are more bespoke – tailored to align with Moonfire investors’ own visions and strengths.
One example, which I gave at Pulse, was Mattias’s hunch that there may be a statistical correlation with success for founders who have worked across several different countries.
Francesco Farina, a Machine Learning Scientist at Moonfire, found that Mattias’ hunch was correct – and this metric was fed into the model.
The fallibility of Founder scores – The process is often one of trial and error – but also of continuous improvement.
The machine is always being improved. We’ve had great founder scores but then discovered that the person is not so great. And awful founder scores, when the founder is great. And these are the really helpful cases – because then we learn something about where our engine is lacking.
All of us at Moonfire add to this process and try to create a hive mind – where we converge on ‘the optimal perspective’ with our contributions.
ML for Managing Funds – Beyond sourcing and evaluation, where AI is also used for competition analysis and investment risk, we use ML to assist us in enrichment, investment, and support too.
We use automation and predictive models to manage our funds more effectively and then help founders to develop some of the same technology.
Doubling down on Sectors – Rather than detract from human-decision making, we see automation as additive to our very thesis-oriented approach.
As Mattias iterated on at Pulse:
“We spend time looking at the sectors that we really care about, and gain conviction even before we’ve met the founders. And then we say – this is what we think is around the corner, and if we see something that’s kind of in that area, we double down on it.”
A Purpose-driven Approach – Moonfire has a strong belief that ML makes its VC fund’s processes more considered and effective. As I said at Pulse:
"Essentially, we are a bunch of scientists working to use technology effectively in this ecosystem – to propose falsifiable hypotheses about how we can introduce better processes – and to scale up the rate at which we make higher-quality decisions. We are trying to make already expert venture capitalists super efficient, super accurate, and super high-throughput in a very consistent, repeatable way.”

Optimising for diversity and fairness in the startup space
If you optimise for performance you can do so at the expense of fairness. And you could optimise for fairness at the expense of performance. Here at Moonfire, we’re trying to optimise for both at the same time.
At Pulse, we discussed how – in terms of startups and innovation – Europe is on fire, with more seed funds needed to build up the whole ecosystem, and more help for founders at the beginning of their journey.
By optimising for greater geographical diversity, together with fairness and performance – we hope to achieve better outcomes across all three.
It is this endeavour – to have greater coverage and to build something that is both unique but also amplifies networks and relationships – that galvanises our team.
As Moonfire becomes more well-known in deal flow across multiple regions, we also appreciate the challenges ahead to further increase opportunities that enable greater racial and gender diversity.
It is in the spirit of these very human drives for the advancement and betterment of our community, that we now outline some of the key insights from the gathering at Pulse, highlighting what we believe will shape the organisations of the future.
Podcast of the Month
Sohn 2022 | John Collison in conversation with Stanley Druckenmiller
This wonderful interview from the 2022 Sohn Investment Conference in New York last week provides a masterclass insight from Stripe co-founder and president, John Collison, who interviews famous hedge fund manager Stanley Druckenmiller. We found it very interesting how the conversation delves into the macro issues around the current economic downturn, and why we may have a bit longer to wait before exiting this recession.
Good Read of the Month
Embedded Finance: When Payments Become An Experience — By Scarlett Sieber and Sophie Guibaud

This recent release is an indispensable and eye-opening exploration of one of the most exciting and influential technologies in development today. Embedded Finance details a revolution in financial services, banking, and technology that has already begun, whilst highlighting an exciting view of how our day-to-day lives will look like in 2030.
Thank you for tuning in. Not yet subscribed? You can sign up on our brand new blog page. If you know someone who’d love to read this newsletter, please feel free to share it. Wishing you a great June!
All the best,
Mike, Mattias, and the Moonfire team.
🌓🔥