Moonshot I: Where the chips fall
The first edition of our AI newsletter: Moonshot. An opinionated digest of noteworthy innovations, thoughts on industry shifts, and updates on our own projects at Moonfire.
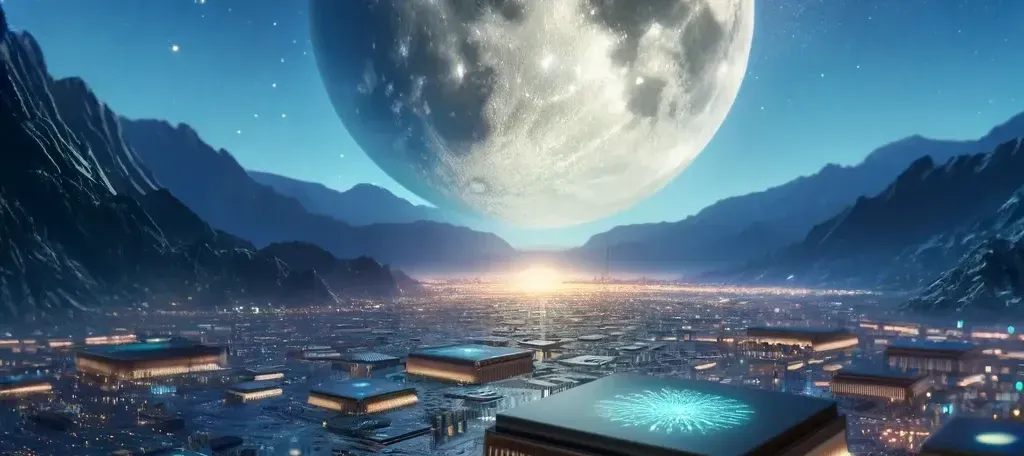
Welcome to the first edition of our AI newsletter: Moonshot.
Working on and with AI every day, we decided we’d curate and share the interesting stuff we find along the way, on a roughly quarterly basis. Our goal is not to provide a comprehensive roundup of AI news, but to provide a more opinionated digest of noteworthy innovations, thoughts on industry shifts, and updates on our own projects at Moonfire.
In this first issue, we’re focusing on the backbone of AI: chips.
Compute hardware is a critical resource when it comes to all kinds of compute workloads, and AI is a very useful, very compute intensive kind of workload. This issue of Moonshot is going to introduce you to a few recent innovations and ideas.
We hope you enjoy it, and let us know what you think.
– The Moonfire engineering team: Mike, Jonas, and Tom 🌗🔥
What's been happening
It’s been a quarter of new chips – from Google and Meta’s in-house semiconductor models to a moonshot “thermodynamic” chip.
- OpenAI released GPT4o. Not a chip, but had to mention it. Any combination of text, audio, and image as inputs and any combination of text, audio, and image as outputs; real-time translation; emotion detection. Obviously, the model has limitations, but it’s a big step in making human-machine interaction more naturalistic. And with the API, we’re going to see a lot of cool stuff being built on it.
- NVIDIA’s Jensen Huang announced the new Blackwell chips. Bigger, better chips. But is that good enough long term for bigger compute and better performance? As Groq puts it, “NVIDIA’s Blackwell isn’t just faster horses, it’s more of them, tied to more buggies, yoked together by an expanding network of harnesses. The scale is stupendous, the engineering remarkable, and, it’s still a horse and buggy architecture.” And that’s not to mention the energy consumption.
- Groq announced its new Language Processing Unit (LPU) Inference Engine chips. They differ from GPU architecture, designed to overcome the typical LLM bottlenecks that choke GPUs and CPUs: compute density and memory bandwidth. This means faster inference for computationally intensive applications like AI, and they use less energy to do it.
- Extropic came out of stealth, building a new type of “thermodynamic” chip. It’s a bit of a moonshot, but an interesting one. It’s based on the following insights: the size of transistors is growing ever smaller, and at some point they’ll become so small that they’ll become “noisy” in the way that quantum chips are. Thermodynamic chips, so Extropic argues, are inevitable by 2030. The practical use case for these chips are GenAI models, which essentially try to estimate a probability function and sample from that function (thereby creating images, words, etc). By harnessing the inherent noise of thermodynamic chips, Extropic hopes to do this much faster and more efficiently. At the same time, because you want a certain element of randomness/noisiness in your chips, you don’t have the problem that quantum computers have: they’re still too noisy for any meaningful work.
What we're thinking
Hardware is the once and future battleground of AI. There are not enough GPUs to train AI, choking acceleration in terms of both dollars and compute.
This is partly a geopolitical issue. 90% of the most advanced semiconductors are produced in Taiwan. A single, fragile point of failure, particularly given China’s contested claim over the country and its tensions with the US. There are other centres of chip production – the US, Japan, South Korea – and Europe is driving to onshore its own capacity, with plants proposed in Germany, France, and Italy, but the cost of production is much higher. However it plays out, AI progress relies on cooler heads prevailing.
But, more fundamentally, it’s a technology problem.
While specialised hardware accelerators – like GPUs and TPUs – have been the main driver of recent AI progress, they may eventually hold the field back. This hardware is based on a circuit called a systolic array, which is well suited to accelerating the matrix multiplication that is common to almost all AI models. The potential problem, however, is that we overly optimise both our hardware, and the entire field of AI, for matrix multiplication – narrowing our focus on innovative model and hardware design.
We need new science when it comes to AI hardware accelerators and silicon-based compute in general. If we want to move AI out of the data centre and into people’s hands, we need chips that can handle modern AI workloads in compact or energy-constrained environments. That’s why moonshots like Extropic’s thermodynamic chip or the DARPA funded attempt to use mixed-signal circuits and architectures for more energy-efficient AI computation are exciting. They’re reimagining traditional Von Neumann architecture and the physics of computing.
What we've been up to
- We recently switched from an Airtable-based CRM to our home-grown investment platform, which gives us a live view of our investment pipeline. It’s been in the works for a few months, and we are really excited about all of the fun LLM-powered features that we have been able to build. We’ll get into the specifics later, but this new platform has a lot of cool new features to help our investment team be more efficient and accurate in sourcing and making investment decisions – as well as providing a much better user experience. And that’s just the start. We’ll continue to iterate on the Moonfire Platform in the coming months, with several exciting, innovative features on the roadmap. Watch this space! 🪐
- Moonfire was recently recognised as one of the top 20 data-driven VCs (i.e. at least one engineer on the team, and have developed at least one piece of internal tooling), out of a list of 190 – and the only firm with more engineers than investors.
Until next time, all the best,
– Mike, Jonas, and Tom 🌗🔥