August '24: AI Hype and Hiring
Differentiation in the AI hype cycle, how to hire engineers as an early-stage startup, and why machines learn.
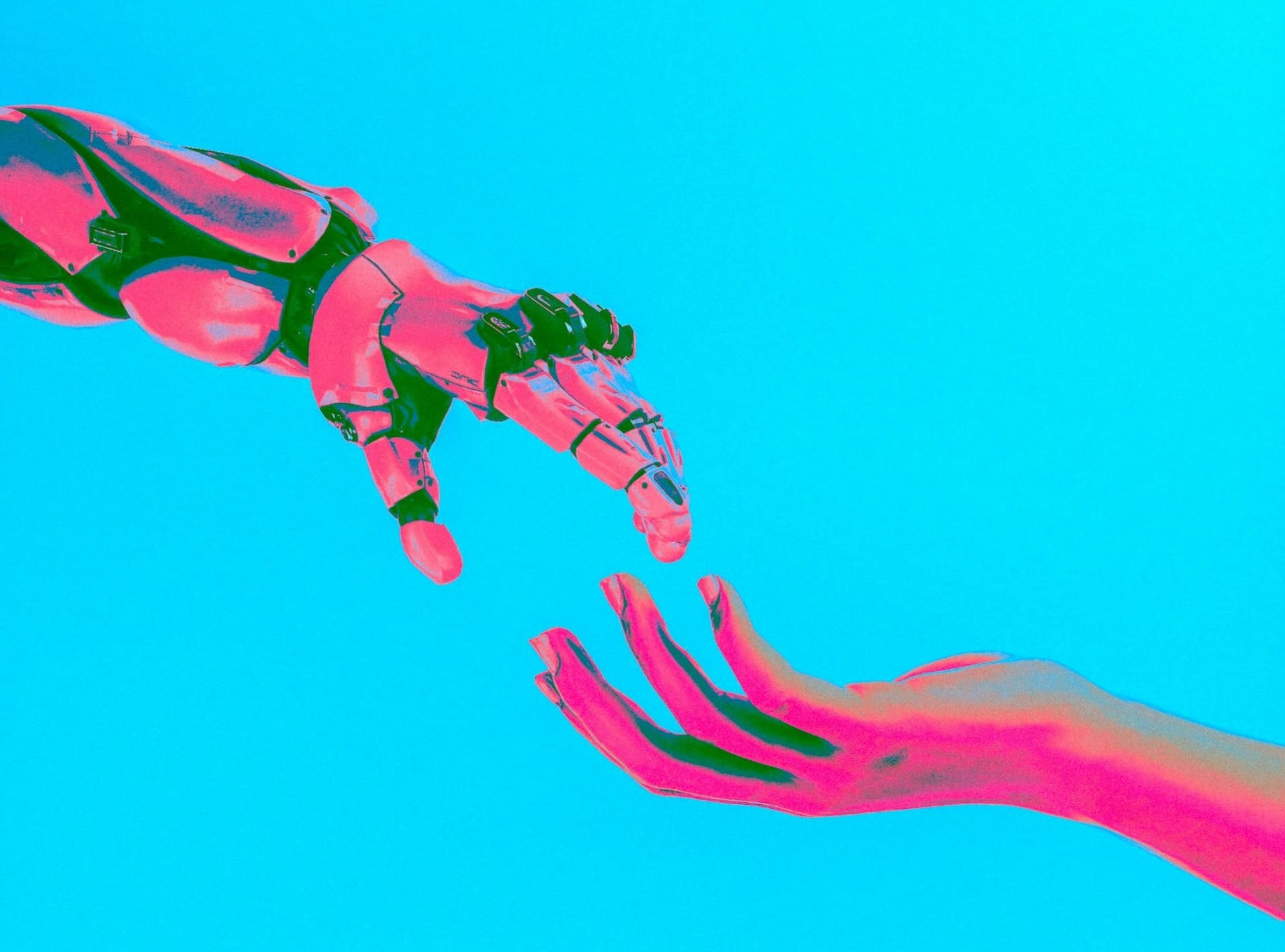
Hello and welcome to the August newsletter.
This month Mike talks about how founders can differentiate themselves amid the hype and uncertainty of AI. As we approach hiring season, we're also re-upping Mike's advice for how early-stage startups should approach hiring engineers.
If you missed it, we asked some of the brilliant entrepreneurs we've invested in about what brought them together with their co-founders, and how their individual skills combine to make a winning team. Watch their answers here.
Enjoy.
Mattias and the Moonfire team
🌓🔥
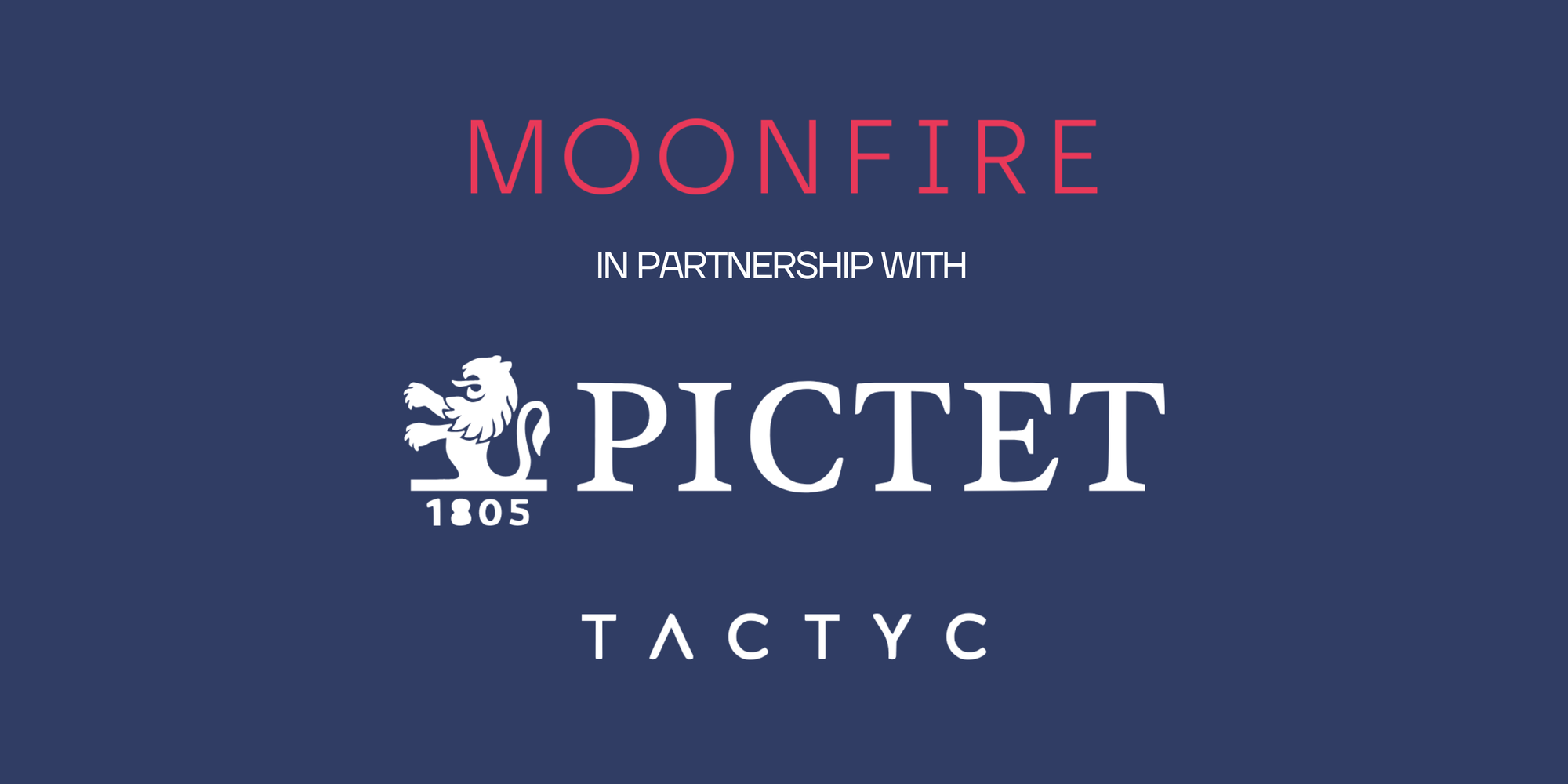
The Snapshot
Here's a quick roundup of cool stuff we saw this month:
- Compact language models via pruning and knowledge distillation.
- What business problems are newly solvable with AI?
- Satya Nadella on leadership – food for founders who like to be ambitious builders.
- What attributes of a market make AI agents / service-as-a-software attractive to pursue?
- How to build a high-end, tech-enabled healthcare business.
- The condensed Marc Andreessen guide to career planning.
What's Up at Moonfire?
How to differentiate in the AI hype cycle
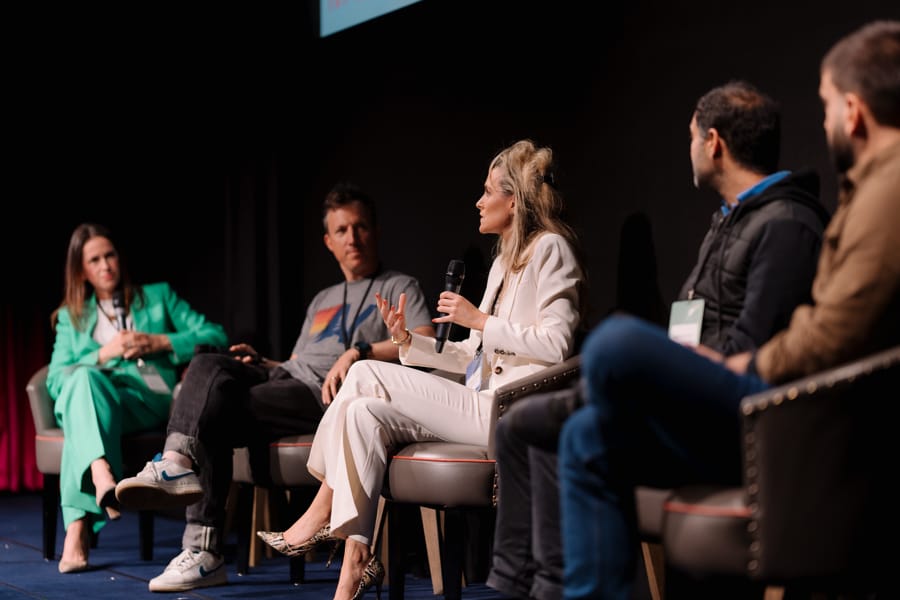
For the first time in software, people are building products and platforms where future progress hinges on scientific progress, not just engineering and product development. But this creates a challenge with our ability to predict progress based on past experiences, because reasoning about the pace and direction of fundamental scientific discovery, as opposed to things that we can build, is hard. It’s all too easy to see the progress we’ve made so far – think GPT3 to GPT4o – and assume it will continue unabated.
How do you, as a startup founder, differentiate yourself amid this hype and uncertainty?
This was one of the topics of a panel at our latest Pulse Summit – with me; Megan Quinn, investor, builder and board director; Mehdi Ghissassi, Director and Head of Product at DeepMind; Fabian Roth, Co-founder and CEO of fore ai; and Rosalyn Moran, CEO of Stanhope AI – and I wanted to explore some of the points that we discussed.
Exploring new architectures and human intelligence
Transformers have been true to their name, dominating the field for good reason. They’re incredibly powerful and have a lot of road left to run, both in terms of model improvement and new use cases.
The risk, however, is that people’s expectations of progress might include things not natively provided by transformers – like memory and active learning. And such misaligned expectations could drive divestment and push us into another AI winter.
That said, there’s already a lot of great work happening to augment the transformer architecture, as well as address some of its limitations, like mitigating the quadratic scaling problem of self-attention to reduce the computational complexity while increasing context sizes.
In parallel, others are exploring approaches that can address aspects of artificial intelligence not natively provided by transformers. Our portfolio company Stanhope AI is drawing inspiration from human intelligence, working from the neural architectures and computational underpinnings of the brain. They are taking the concept of active inference from neuroscience into AI as the foundation for software that will allow robots and embodied platforms to make autonomous decisions like the human brain.
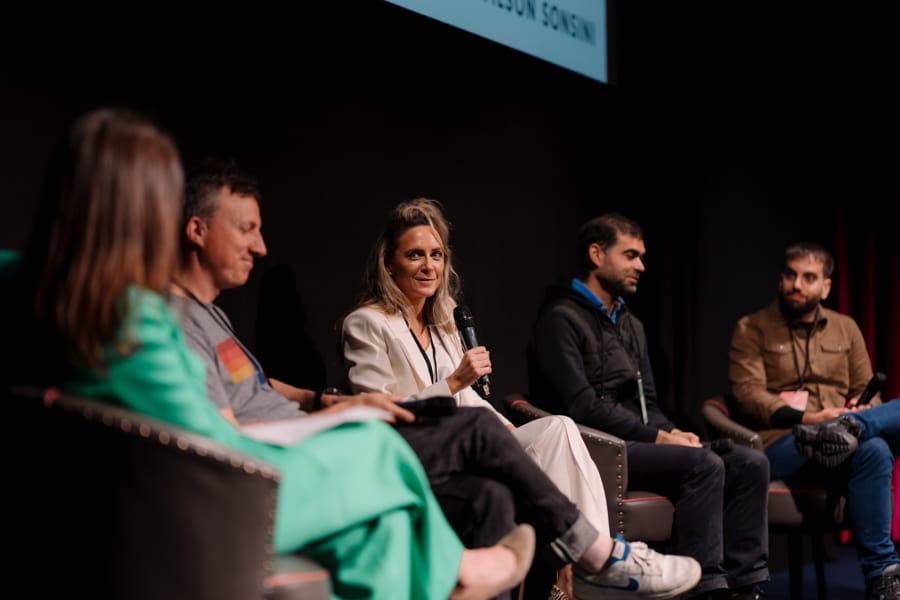
The idea is not to replace transformers, but to complement them. As Rosalyn explained, while deep learning is effective for tasks like image generation or MRI analysis, applications requiring reasoning about dynamic environments, such as autonomous systems, could really benefit from human-like intelligence.
Getting the basics right
But progress and differentiation in AI also involves getting the basics right. ‘You’ve got to walk the walk, and build specific applications that are actually bringing value to what you’re doing,’ said Fabian. Companies need to be willing to invest the time and resources to bring AI prototypes to production quality.
As Fabian noted, a trustworthy solution built with transformers requires trustworthy, accessible data to reason on and function effectively. A lot of value can be created just by getting the plumbing and integration right. ‘I think being able to glue together APIs from different applications on your phone or computer and automate that with natural language is going to be extremely powerful very soon.’
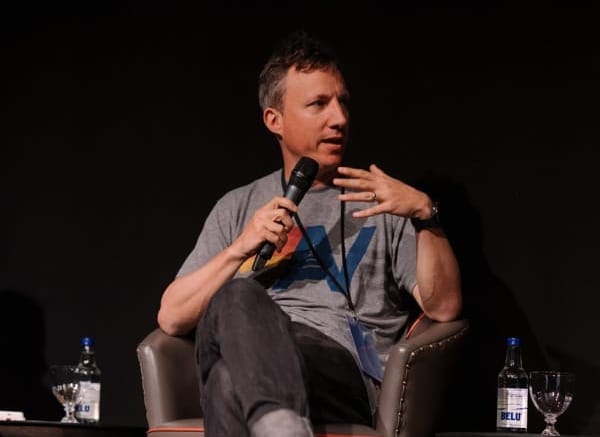
Really, the key to differentiation in AI is timeless: build products that people want. Start with the user and their needs, not the technology. That doesn’t mean you need to solve a “hair-on-fire” problem. Look at ChatGPT, the fastest growing consumer application in history. It didn’t address a hair-on-fire issue at launch and, as the next big thing so often does, it feels like a toy. But giving people the ability to rapidly extract high-throughput information is as much of an important problem as has ever existed.
However, a big difficulty right now is predicting where the big players are going with the technology. A lot of startups are building on top of these platforms, only to have their lunch eaten. ‘As a startup, you need to solve a very specific problem and find the right business case around it, that is specific enough that it’s not going to be solvable by a generic system,’ advised Fabian.
Thinking ahead
It’s also about thinking ahead – envisioning the new ways of doing things that AI can enable or augment. ‘As these things develop and you improve latency, cost, quality, [...] there will be things that we just can’t think of now,’ said Mehdi. ‘Think about Google Maps: Uber was only possible because of Google Maps.’
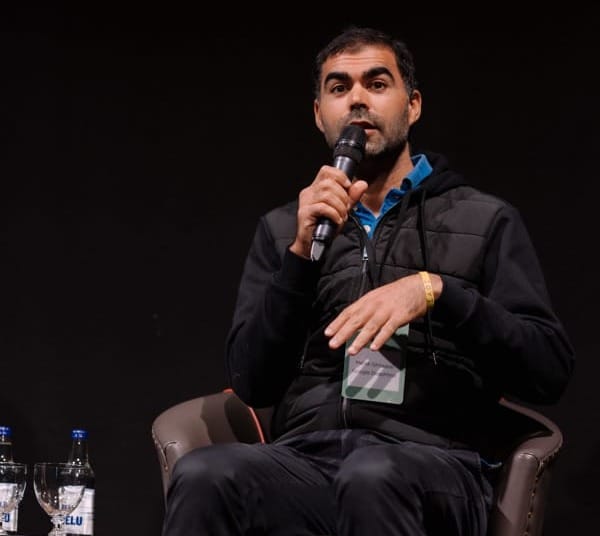
This means thinking carefully about the composition of your founding team. You need at least one person who understands and has good, practical experience in the field. ‘If you’re not able to have someone in the team who has that muscle, it’s going to be hard to know what’s really possible,’ said Mehdi. You also need to understand the specific user needs, workflows and risks of the vertical you’re going after, and adapt your team’s expertise to it.
Right now, LLMs can be applied to more creative, administrative, and financial tasks if you’re willing accept the risk of errors, but areas like healthcare, defence, or any case where human lives are at stake, are much riskier; if you predict something wrong, the cost is fatal.
We need people building in these high-risk spaces, but you need a deep understanding of the risks, and know how to mitigate – or ideally avoid – them. Be prepared for a longer, more challenging path, even though the risks will make it a less crowded market.
Seeing the future
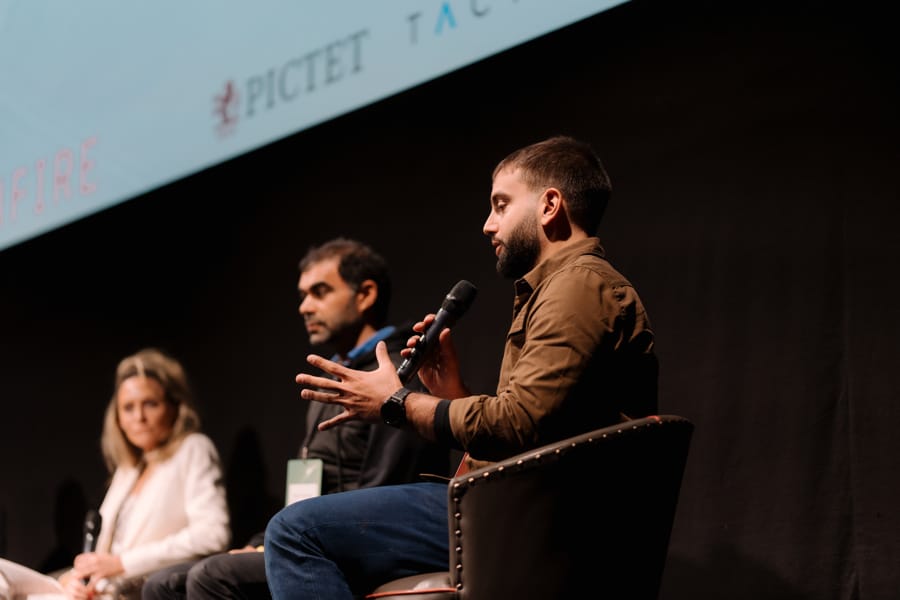
Differentiation is not just within the technology itself, but how you apply it to a given product domain in a differentiated way to do something that hitherto wasn’t possible. You need to really think critically about what new fundamental cognitive capabilities you have available to you, what they’re useful for, and how you can apply that to the domain you’re working in to actually create a differentiated experience that’s useful for different people.
Mehdi captured it nicely: ‘If you’re building a company now, and you’re in it for the next decade, in five years some of the limitations will have been solved, and you’ll benefit from that. It’s a hard balancing act: how much risk do you take and how do you see the future? Perhaps you should double down on the view of the world that you have and that you think is unique.’
– Mike
Hiring
Early-Stage Hiring for Engineering Teams
My advice from a couple of years ago on how early-stage startups should approach hiring engineers is still relevant here.
I've received feedback from folks saying that they think it's a surprisingly involved interview process for a startup, but I think that's exactly what you need, especially for senior engineering hires early on who have the potential to be very influential and move into CTO, VP roles.
Part I
In part I, I talk about how to establish a robust, repeatable process that balances technical assessment with cultural alignment. The key is to design an interview loop that is both rigorous and respectful of candidates’ time, ensuring flexibility to accommodate varying profiles. By focusing on these principles, you can build an engineering foundation that supports your long-term vision.
Part II
In part II, I break down each step of the technical assessment process. I outline how to effectively evaluate candidates’ coding skills, problem-solving abilities, and technical depth through structured interviews and take-home challenges. The goal is to identify engineers who not only meet the technical requirements but also align with your team’s culture and values. That's how you build a team that’s both technically sound and cohesive.
– Mike
Podcast of the Month
The Social Radars: Replit Co-Founders, Amjad Masad & Haya Odeh
Great episode with the co-founders – and husband and wife – of coding platform Replit. Amjad and Haya talk about the early days and the vision they couldn't let go of: bringing coding to everyone.
Good Read of the Month
'Why Machines Learn: The Elegant Maths Behind Modern AI' by Anil Ananthaswamy
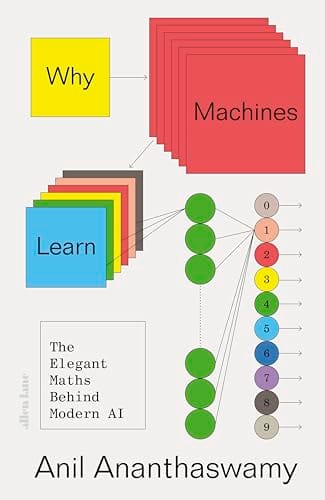
The current AI revolution is founded on mathematics that dates back centuries. The groundwork was laid long ago, but it took the rise of computer science and 1990s video game chips to drive the latest wave. By demystifying the maths behind AI, Anil reveals that both natural and artificial intelligence may share the same fundamental rules. To realise AI's full potential, we need to understand that shared heritage – and the potential limits set by that mathematical foundation.
That’s all for this month.
Until next time, all the best,
Mattias and the Moonfire team
🌓🔥